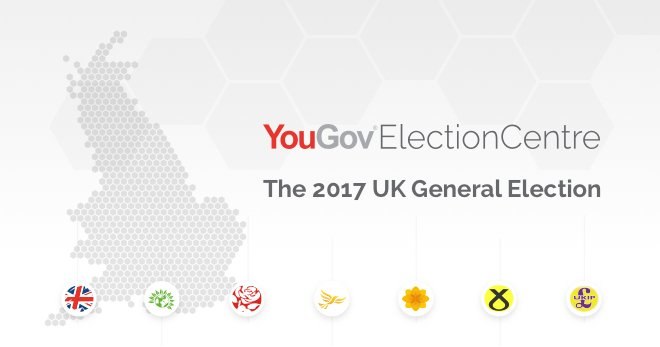
How the YouGov model for the 2017 General Election works
Doug Rivers, YouGov's chief scientist, sets out how YouGov's 2017 General Election model works
Every day YouGov interviews approximately 7,000 panellists about their voting intentions in the 2017 General Election. Over the course of a week, data are collected from around 50,000 panellists. While this is a much larger sample than our usual polls, the samples in each of the 650 Parliamentary constituencies are too small (on average, only 75 voters per constituency per week) to produce reliable estimates.
In the 2016 EU Referendum, in the 2016 US Presidential election, and again in the 2017 UK General Election, YouGov is using a recently developed technique called Multilevel Regression and Post-stratification (or 'MRP' for short) to produce estimates for small geographies (local authorities for the EU referendum, states in the 2016 American Presidential election, and Parliamentary constituencies for the 2017 General Election).
The idea behind MRP is that we use the poll data from the preceding seven days to estimate a model relating interview date, constituency, voter demographics, past voting behaviour, and other respondent profile variables to their current voting intentions. This model is then used to estimate the probability that a voter with specified characteristics will vote Conservative, Labour, or some other party. Using data from the UK Office of National Statistics, the British Election Study, and past election results, YouGov has estimated the number of each type of voter in each constituency. Combining the model probabilities and estimated census counts allows YouGov to produce a fairly accurate estimate of the number of voters in each constituency intending to vote for a party on each day.
It is important to understand the limitations of the model results. First, they are estimates of current voting intentions, not a forecast of how people will vote on 8 June. Panellists tell us how they intend to vote, but they may change their minds and we do not attempt to quantify this uncertainty. Second, the samples in each constituency are too small to be reliable by themselves and are subject to more than just sampling error. To compensate for small sample sizes, we rely on a model that pools data across constituencies. This uses data from panellists who live in other constituencies to augment the small number of actual interviews conducted in a constituency. The model is based on the fact that people with similar characteristics tend to vote similarly, but not identically, regardless of where they reside. While this has worked well in the past (our MRP model in the 2016 EU Referendum consistently showed that more voters favoured leave than remain, and that Hillary Clinton would win the popular vote in the 2016 US Presidential election by a narrow margin, but that midwestern battleground states were too close to call), models cannot produce estimates as accurate as a full scale poll in each constituency.
Using MRP, we have classified constituencies as safe, likely, or leaning to a party or as a toss-up. The displays for each constituency provide a vote estimate for each party and a 95% confidence interval. These are the model's best guess of what a large poll would show if it were conducted in that constituency on the same day. Readers should focus on the confidence intervals as giving a more reliable estimate of current voting intentions. Even these are not fail-safe: we would still expect the interval to be wrong in 30 to 40 constituencies.
The model was developed primarily by Professor Ben Lauderdale of the London School of Economics in conjunction with YouGov's Data Science team, headed by Doug Rivers of Stanford University. The data are streamed directly from YouGov's survey system to its Crunch analytic database. From there, the models are fit using Hamiltonian Monte Carlo with the open source software Stan. Stan was developed at Columbia University by Andrew Gelman and his colleagues, with support from YouGov and other organisations. YouGov will be updating the model estimates on a daily basis.
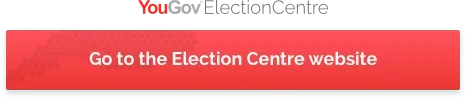